Michael S. Watt
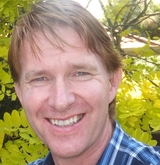
Contact
- Phone: +64 3 363 0937
- Email: Show email
- Web: http://nz.linkedin.com/pub/michael-watt/76/b4/7a3
- Team: Data and Geospatial Intelligence
- Role: Portfolio Leader, New Value Digital Forests and Wood Sector
About
Dr Michael Watt is a Principal Researcher who specialises in remote sensing and development of models to characterise growth, health and wood quality of the forest resource. He has published over 170 refereed papers around these and other topics within forestry. Michael works extensively with the New Zealand forest industry and has a track record of undertaking science that has been adopted by the industry. He is co-leader of the Remote Sensing cluster group which is one of the most well attended industry focus groups within the forestry sector. Michael has many international links with other research groups in Europe, Australia and South America.
Qualifications
- PhD (Forestry), University of Canterbury, New Zealand -2003
- MForSc (with distinction), University of Canterbury, New Zealand -1999
- BForSc, University of Canterbury, New Zealand -1995
Research capabilities
- Use of remote sensing technologies to characterise the forest resource
- Effect of climate change on forest productivity
- Models of tree growth and wood quality
- Modelling the spatial distribution of pests
- Tree physiology
- Most aspects of weed science
Career highlights
- Science New Zealand – Team Award for Geomatics Team 2020
- Forest Owners Association award for Science of International Quality 2019
- Roger Newman Science Excellence Award 2016
- Future Forest Research Award for innovation that enhances sector value, 2011
Selected papers
Watt, M.S., Kimberley, M.O., Rapley, S., Webster, R. (2021) Comparing volume productivity of redwood and radiata pine plantations in New Zealand. Forest Ecology and Management 500, 119628.
Watt, M. S., Buddenbaum, H., Leonardo, E. M. C., Estarija, H.J., Bown, H.E., Gomez-Gallego, M., Hartley, R., Pearse, G.D., Massam, P., Wright, L., Zarco-Tejada, P.J. (2020) Monitoring biochemical limitations to photosynthesis in N and P limited radiata pine using plant functional traits quantified from hyperspectral imagery. Remote Sensing of Environment 248, 112003.
Pearse, G. D., Tan, A.Y.S., Watt, M.S., Franz, M.O., Dash, J. P. (2020) Detecting and mapping tree seedlings in UAV imagery using convolutional neural networks and field-verified data. ISPRS Journal of Photogrammetry and Remote Sensing 168, 156-169.
Leonardo, E. M. C., Watt, M. S., Pearse, G. D., Dash, J. P., & Persson, H. J. (2020) Comparison of TanDEM-X InSAR data and high-density ALS for the prediction of forest inventory attributes in plantation forests with steep terrain. Remote Sensing of Environment 246, 111833.
Watt, M.S., Kirschbaum, M.U.F., Moore, J.R., Pearce, H.G., Bulman, L.S., Brockerhoff, E.G., Melia, N. (2019) Assessment of multiple climate change effects on plantation forests in New Zealand. Forestry 92(1), 1-15.
Pearse, G, Dash, J. Persson, H., Watt, M.S. (2018). Comparison of high-density LiDAR and satellite photogrammetry for forest inventory. ISPRS Journal of Photogrammetry and Remote Sensing 142, 257-267.
Dungey, H. S., Dash, J. P., Pont, D., Clinton, P. W., Watt, M. S., & Telfer, E. J. (2018). Phenotyping whole forests will help to track genetic performance. Trends in Plant Science 23(10), 854-864.
Pearse, G., Watt, M.S, Morgenroth, J., Dash J. (2017) Optimising prediction of forest leaf area index from discrete airborne lidar. Remote Sensing of Environment 200, 220-239.