Deep learning algorithm can identify seedlings
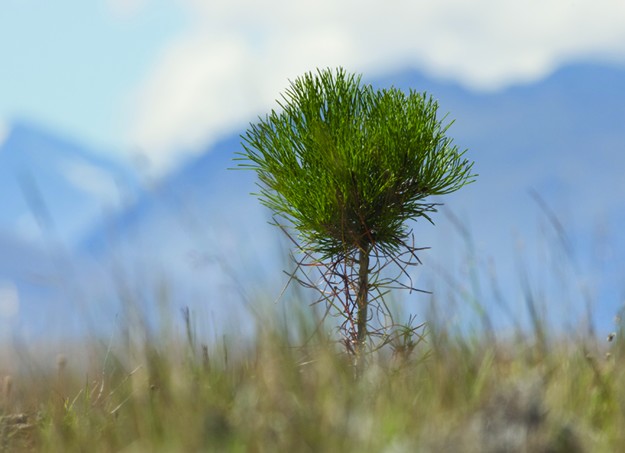
Scion has pioneered a new artificial intelligence (AI) based system that uses low-cost RGB imagery from UAVs (unmanned aerial vehicles) to identify radiata pine seedlings. The algorithm they have created could soon replace manual surveys, which are laborious, sometimes dangerous and only suited to small areas.
Surveys are traditionally carried out after planting to ensure the right number of trees have been planted and survive to reach the target for each site. Scion’s advanced AI-system can detect seedlings with extremely high accuracy, detecting between 94-98 per cent of seedlings in a range of test sites and landscapes.
The next steps are to trial the method with industry partners and explore expanding the algorithm to detect native tree species.
All afforestation efforts in New Zealand stand to benefit from this work as it unlocks the potential for large-scale automatic assessments of newly planted sites for density and survival.
“This work is a highly innovative combination of drone imaging and machine learning technology. It opens up entirely new possibilities for forest and land management - monitoring seedlings is only a start.” - Prof. Dr. Matthias Franz University of Konstanz
The team has also developed a data processing procedure that takes large volumes of UAV imagery and transforms it into a format used in deep learning models without losing spatial information. They have also amassed a substantial dataset for models to ‘learn’ how to tell tree seedlings apart from weeds and other objects across a range of conditions. The original deep learning models were intended for use with mobile phone photos and the team has now adapted them to rapidly handle large datasets using Scion’s state-of-the-art ‘GPU’ infrastructure.
Working with both Forest Growers and Te Uru Rākau, project leaders will shape proposals for the next stage where we refine the approach to integrate the model into existing workflows. Future planning will include developing a more complex model suitable for use at low-cost and with largely automatic quality assessments. We also plan to expand the model to detect other species including natives to identify seedling mortality.
All afforestation efforts in New Zealand stand to benefit from this work as it unlocks the potential for large-scale automatic assessments of newly planted forest sites for density and survival.