Growing forest productivity using remote sensing and data science
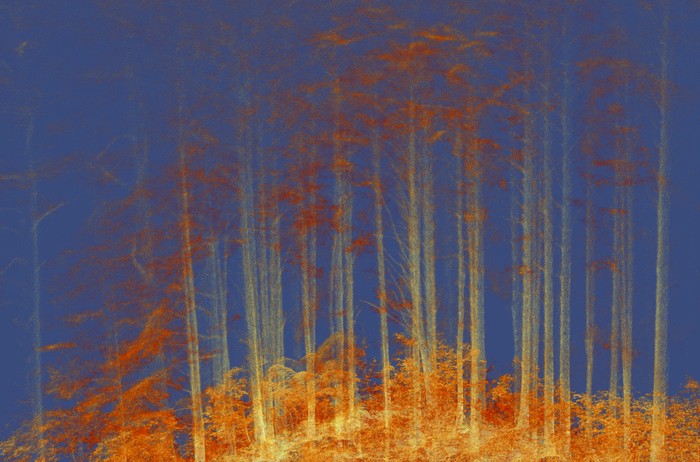
Understanding the drivers of productivity is essential for forest managers. A forest phenotyping platform has been developed that allows forest managers to do just that.
The platform is a group of technologies that form a base to create new and meaningful information about genetics, environment and site. Scion’s phenotyping platform includes forest management data, remotely sensed data from whole forests, machine learning and modelling approaches.
Using this platform, Scion has determined that the underlying genetics of a forest stand is one of the major factors that underpins the stand’s productivity.
The remotely sensed data within the platform can also be used to identify individual high-performing trees in a forest. This is a unique and important tool that could help optimise tree management and select resistant trees in the event of a major pest or disease incursion, for example.
Results also show that forest productivity can be improved by identifying and breeding more of the best performing trees and eliminating the worst performing trees.
This tool has already influenced how forests are managed in New Zealand, and the concept can be applied to smaller forests using available forest inventory data.
Phenotypes are an accurate and precise description of an organism and are influenced by our genes and environment. In plant breeding, understanding how characteristics are affected by genes and environment can speed up genetic improvement programmes.
However, measuring mature trees in a forest is challenging. Trees are large and difficult to measure accurately, there are operational limits in the number of trees and sites that can be measured and separating genetic effect from environmental variation is not straightforward. The challenges of characterising individual trees are restricting current tree breeding and our understanding of the relationships between genetics, environment and site.
Recent technological advances in remote sensing, genomics and layers of geographic information system (GIS) data such as climate, soil type and forest management activities can be combined to relieve the phenotyping bottleneck and create the phenotyping platform.
The research team worked with Kaingaroa Timberlands Ltd who supplied the remote sensing and forest records for Kaingaroa Forest to use as a model to develop the concept and show its potential for maximising genetic gain in forests.
Focusing on areas of radiata pine with the largest amount of recorded genetic information and forest management history, remote sensing was used to model phenotypic traits such as tree height, and diameter. Productivity was calculated from canopy height derived from LiDAR data. Then layers of GIS data such as climate, soil information and stand management were added. An additional machine learning model was then implemented to extract the key drivers of productivity, estimate the productivity on missing sites, and predict the influence of favourable clones on the productivity across the whole forest.
Initial indications from the prototype phenotyping platform are that genetics and climate had the strongest influence on the productivity of the trees in the study. Analyses at the individual tree level have also quantified the effects of competition on tree growth, highlighting the importance of good management.
The overall aim of a phenotyping platform approach is to increase forest productivity and sustainability by matching trees with improved genetics to the environment they will be growing in and tailoring the management regime to fit. This precision-based approach is the foundation of future forest planting that will ensure the right tree goes in the right place, with the right purpose and management to realise the best outcomes.