Managing newly planted forests and indigenous biodiversity with AI
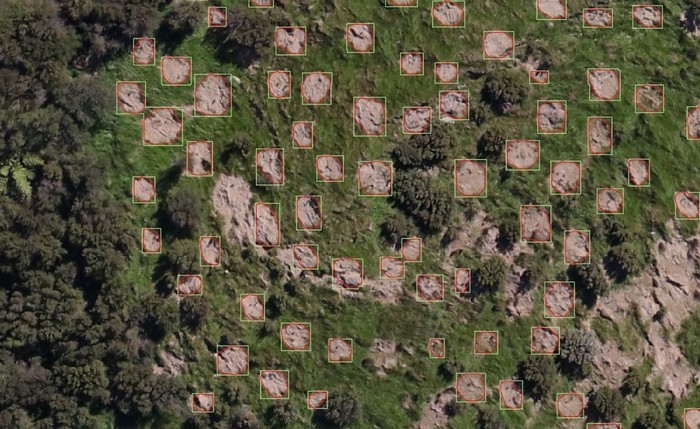
Scion is working with remote sensing specialists Indufor on an artificial intelligence-powered model that uses remotely sensed data to help manage newly planted radiata seedlings and indigenous biodiversity.
How it works
Together, Scion and Indufor have created a process that can transform remotely sensed data into a model to identify radiata seedlings and pockets of indigenous forest. This data can be used to understand seedling survival rate, while also gaining a clear picture of the indigenous biodiversity on site.
High-resolution aerial imagery (5 cm) is processed through a trained deep learning model, which then identifies, maps and draws polygons around the newly planted radiata seedlings and pockets of indigenous forest.
The new model offers many advantages. Unlike earlier models, it needs much less data to train it. The approach is also much lower cost per unit area than UAV-based imagery and has been successfully used over large areas of afforested land. The team is now exploring the potential to use the 3D point cloud generated alongside the aerial imagery during the data generation process. The point cloud data could potentially be used to characterise the biomass, height and density of the indigenous forest identified on each site.
Undestanding biodiversity
Indufor is using this model now to help foresters map the exotic and indigenous species growing on their land, and they have seen a great demand for this type of service. In addition to assisting foresters to monitor the establishment of their newly planted radiata seedlings, it aids them in identifying indigenous forests on their land and how to integrate them within the landscape appropriately, e.g. seeing how big and far apart forest fragments are and what steps could be taken to join them up.
Mapping also helps answer key questions about the biodiversity carrying capacity of the indigenous forest, for instance, can the existing fragments support indigenous bird species that help spread tree seeds? Knowing this could help land managers to understand what activities are required to help their pockets of indigenous forest to expand and connect.
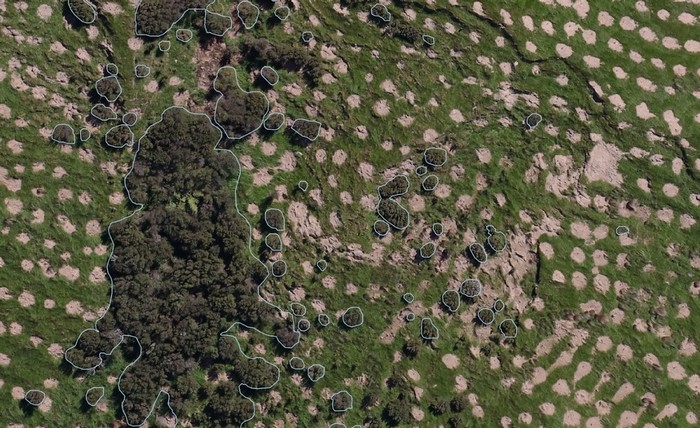
The future
With more development, the team hopes to update the model to identify the indigenous species mix within a stand, painting a much richer picture of the biodiversity and biomass. This capability would help answer long-term planning questions, such as, is there a good mix of tall woody species and shrubs on the land? Do I need to carry out predator control and plant native species to increase the value of the existing forest?
Scion project lead Dr Grant Pearse says, “There’s a lot in favour of this model; it’s cost-effective, scalable and accurate. We hope that with a little more development we could accomplish a lot more”.
Dr Pete Watt, leader of Indufor’s resource monitoring team, says, “We are excited to leverage this promising technology and further explore how we can use it to accurately map planted and native forests across landscapes”.
Forests, both native and exotic, are vital to New Zealand’s long-term climate change response, and this technology could play a role in ensuring those efforts are successful.