Transforming tree phenotyping
Seeing the forest for the trees: Transforming tree phenotyping (TTP) for future forests is a five-year (October 2021-September 2026) research programme funded through the Ministry of Business, Innovation and Employment (MBIE) 2021 Endeavour Fund, with significant in-kind co-funding from Radiata Pine Breeding Company (RPBC) and Lake Taupō Forest Management (LTFM).
Forest-scale phenotyping (characteristics) of millions of trees will enable forest growers to optimally match different tree genotypes to site conditions under current and future climates, increasing indigenous and exotic plantation productivity, and forest health and resilience.
Scion, through the TTP programme, hosted ForestSAT 2024 in Rotorua in September 2024, marking the first time the conference has been held in Australasia. ForestSAT, renowned as the premier global remote sensing conference, offers a pivotal platform to showcase the TTP programme and its milestones, as well as foster collaborations with over 300 international and national specialists. Opened by Rotorua Mayor, Tania Tapsell, the conference welcomed delegates from NASA and featured keynote speakers Dr Crystal Schaaf, a Professor at the University of Massachusetts Boston, and Pablo J. Zarco-Tejada, a Professor at the University of Melbourne. Five scientists from the TTP programme presented their research; read more here.
Benefit to New Zealand
Increases in forest productivity and afforestation resulting from this research strongly support the net-zero 2050 goal. A business case showed that the optimisation of genotype deployment could significantly improve flows of timber and carbon, increasing the net present value of plantations by over NZD$1 billion. The successful development and application of methodologies outlined in the programme will make precision forestry a reality. Cultural phenotyping will strongly encourage afforestation by Māori landowners.
Upcoming events
SilviLaser 2025 - September 2025, Quebec, Canada
Contact
Dr Michael Watt, Principal Scientist
Key findings and benefits to date
The key findings and benefits highlighted below underscore significant strides in advancing tree phenotyping and precision agriculture within the TTP programme.
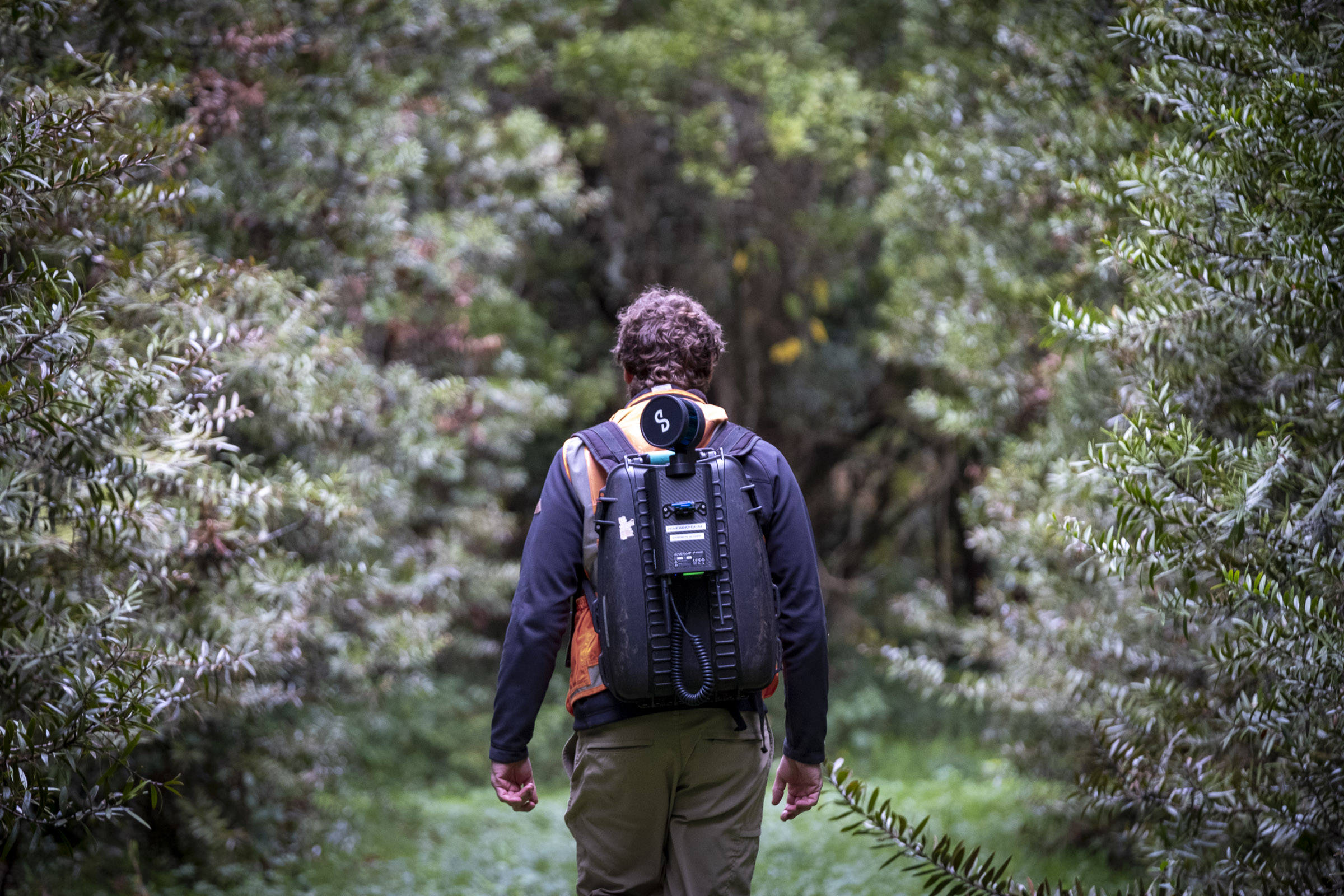
Background
Planted forests are an essential component of New Zealand’s transition to a carbon-neutral bioeconomy. However, our ability to grow radiata pine and other species successfully is at risk due to uncertainty around our changing climate.
One approach to increase our planted forests’ productivity is to use a tree’s phenotype, a product of the interaction between genetics and the environment, to identify trees that grow well in specific environments.
Research into identifying trees with outstanding phenotypes has already begun, but there is more to be learned about our planted forests. New ways of collecting and analysing large scale data that describe important structural traits such as tree volume, form, and carbon sequestration will enable us to rapidly identify exceptional trees. We will combine this information with predictions of key internal traits such as resistance to disease and drought, which will be characterised using hyperspectral and thermal data.
These new methodologies will deliver high throughput forest phenotyping using remotely sensed data and advanced concepts in data science. Combined with genomic data, we will be able to select and breed trees with desirable traits such as high carbon storage and resistance to disease and drought.
The programme also extends to indigenous forests, with the aim of combining data with mātauranga Māori to explore the cultural linkages Māori have to forests and taonga species. This “cultural phenotyping” is expected to lead to modern applications of traditional forest-based economic opportunities including diverse forests capable of delivering a wider range of benefits and to the reinvigoration of Māori customary practices.
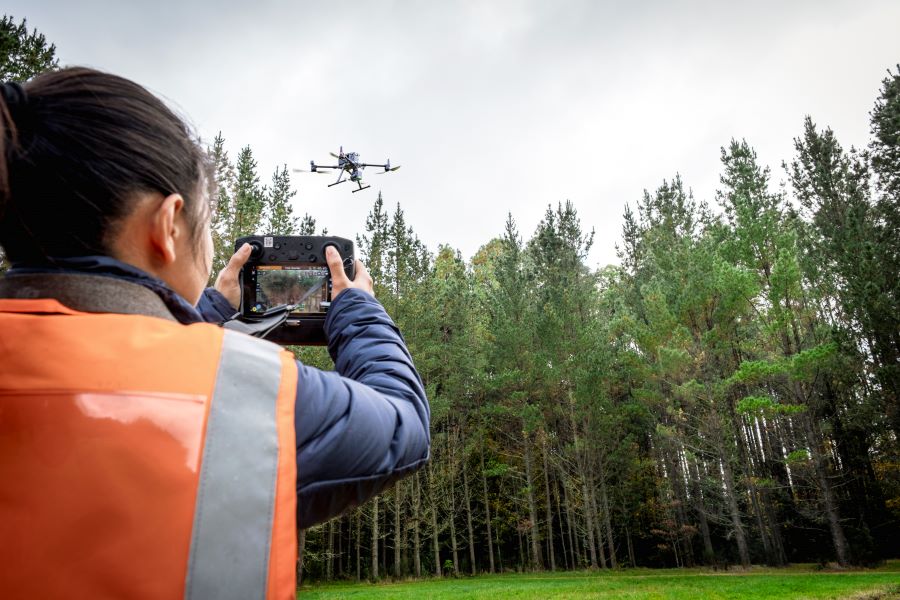
About the research programme
This research initiative encompasses four key areas focused on revolutionising forestry practices through advanced technologies and indigenous perspectives. Furthermore, the programme integrates Vision Mātauranga principles to guide its research efforts.
The 3D characterisation of individual trees using deep learning
This research aims to tackle challenges related to preprocessing point clouds acquired in forestry contexts, aiming to extract fine-grained characteristics from individual trees. We'll validate and deploy methods for capturing and aligning ultra-high-density 3D data using diverse sensors across different forest types. Additionally, we'll develop tools for characterising trees within large 3D forest scenes and we'll innovate new methods for generating synthetic 3D datasets at the tree level to facilitate the training of deep learning models.
Contact: Robin Hartley, Geospatial Scientist
This research area has been investigating data capture methodologies to assess the optimal ways of collecting 3D data to phenotype trees. In this image, an individual tree was extracted from a UAV laser scanner (ULS) point cloud of a breeding trial. The tree was subsampled as part of an experiment to determine what the minimum point density was to be able to predict DBH, height and tree volume before accuracy of the measurements starts to drop. This information will be used to aid in optimising flight planning for UAVs, to make data capture times and data sizes more efficient.
Development of methods to utilise remotely sensed data at the tree level
The research aims to develop methods, using hyperspectral and thermal data, to characterise phenotypic traits related to disease susceptibility and drought tolerance for a series of trials in New Zealand. Combining these data with 3D structural attributes (RA1) will enable the extraction of highly detailed individual phenotypes to be combined with climatic and novel genotypic data (RA3). The application of these methods will be expanded to support cultural phenotyping in complex, indigenous forests (RA4).
Contact: Russell Main, Senior Spatial and Remote Sensing Scientist
Hyperspectral and thermal images captured over a Dothistroma Needle Blight infected radiata pine stand. Both sensors have been shown to be sensitive to stress induced changes in the trees physiology. Severely infected trees appear reddish-brown in the hyperspectral image (left) and exhibit warmer canopy temperatures (pink-yellow) than mildly infected trees (blue-black) in the thermal image (right). The inset graph illustrates the variation in visible near infrared reflectance (VNIR) between mild (green) and severely infected (red) trees. Mapping the disease severity across the stand and correlating it with genotypic information may provide insights into genetic resistance of different tree genotypes. The two sensors have also been shown to be sensitive to pre-visual effects of disease, which could offer rapid, scalable, and early assessments of forest health, ensuring timely and targeted responses to disease outbreaks.
Genetic analysis of adaptive trait data and climate-based deployment
To address the challenge of climate change we will (1) integrate state-of-the art approaches in tree physiology, genomics, climate data science, remote sensing, and geospatial modelling to characterise key adaptive traits (i.e. drought resistance and vegetative phenology), (2) use an unprecedented data set in radiata pine to relate tree ancestries to climates and adaptive traits and (3) develop climate-based seed zones and deployment guidelines for radiata pine and at least one indigenous species in New Zealand.
Contact: Dejan Firm, Forest Ecology and Management Scientist
High precision dendrometers used for continuously monitoring water deficit and radial growth of tree seedlings exposed to drought.
Ngā Mata o Tāne (great forest of many eyes)
To create tools that capture the different ways Māori see and value forests, enabling a distinctive Māori forestry identity to express itself with new economic platforms that also serve to offset the carbon footprint of New Zealand.
Contact: Lania Holt, Research Scientist
Vision Mātauranga
Vision Mātauranga is a key part of what we will do, how we will do it, and the impact we seek to enable a distinctive Māori forestry identity to express itself with new economic platforms. Our research area of Ngā Mata o Tāne (great forest of many eyes) is Vision Mātauranga centric, and it’s strongly connected to all the other research areas in our programme under the themes of:
Indigenous Innovation by creating Māori algorithms and datasets that will synthesise Māori knowledge with remote sensing applications to, for example, be able to depict the distribution of culturally important species.
Taiao aims to change how we analyse the interaction between genetics and the environment, particularly for indigenous species. This shift will help Māori invest in forests that provide various advantages such as biodiversity, lasting impact, and new opportunities. It will transform landscapes, especially where Māori-owned land is not being fully utilised.
Mātauranga Māori by researching modern applications of traditional forest-based opportunities that not only lead to economic opportunities but can also reinvigorate Māori practices and Māori cultural linkages to forests.
Funders and collaborators
New Zealand
- Cucumber
- Defence Science & Technology
- Forest Growers Research
- Interpine Innovation
- Lake Rotoaira Forest Trust
- Lake Taupō Forest Management
- Manaaki Whenua – Landcare Research
- PF Olsen
- Radiata Pine Breeding Company
- SKYCAN
- Survey Solutions Limited
- Te Roroa Commercial Development Ltd
- Te Uru Rākau – New Zealand Forest Service
- Timberlands Ltd
International
Publications
Over the first three years of the programme 11 papers have been accepted for publication. Stay tuned for works in progress; additional publications will be added as they become available.