Identifying pōhutukawa for myrtle rust response
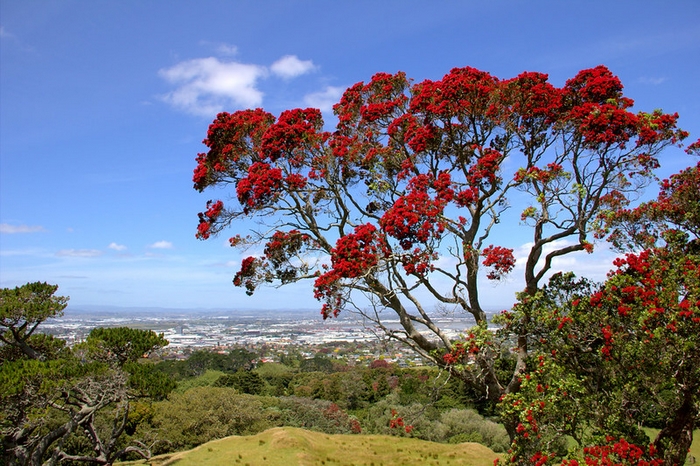
Part one of our feature on Scion’s recent advances in deep learning technology.
Deep learning is a process where computers ‘learn’ to recognise complex patterns by looking at thousands of examples, reaching human-level accuracy for some tasks. The basis of deep learning technology has been around for decades, but it has been limited to theory more than practice because computer processors have not been able to keep up. That is not the case anymore. Huge increases in modern computational power, some nifty mathematical tricks and large datasets have enabled deep learning technologies to thrive and be integrated into our everyday lives.
At Scion, we see huge potential for deep learning to make unprecedented gains in many areas of our work. We are embracing deep learning in biosecurity, forest inventory and phenotyping, and forestry genetics. The following examples from our work with deep learning are showing promise across a variety of fields.
Biosecurity benefits
Part of the response to any biosecurity incursion in New Zealand involves mapping and identifying the host species of the pest or pathogen. This is a standard part of managing the incursion and helps managers to predict where the pest or pathogen might show up next.
When the myrtle rust plant pathogen was first found on the New Zealand mainland in May 2017, there was immediate concern for our 37 native myrtle species, as well as the introduced myrtles. One of the most precious species at risk is the pōhutukawa (Metrosideros excelsa).
The pōhutukawa is highly valued culturally and an iconic feature of many kiwi beaches. It also grows as an amenity species in Australia, where observations showed that the species is susceptible to myrtle rust.
The conventional next step after an incursion is to send hordes of trained personnel to infection sites to locate host trees and inspect them. This is a difficult task to complete on a large scale. Trees can be spread across a mixture of public and private property or in hard-to-access areas. Carrying out large-scale searches is also very costly and time consuming.
Deep learning models overcome many of these barriers. They show incredible potential for fast, large-scale tree species identification using aerial imagery with only three colour channels (RGB) the same type of imagery your mobile phone can capture.
Scion researchers Drs Grant Pearse, Alan Tan and colleagues have found great success with deep learning technologies. With support from the Ministry for Primary Industries (MPI) they have put this technology to work looking for pōhutukawa.
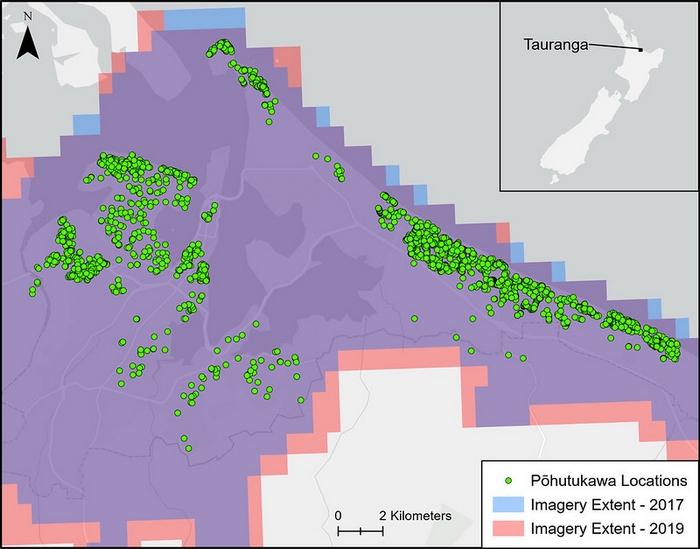
Searching for pōhutukawa
Traditional tree detection models often use lidar and multi-spectral imagery to capture features specific to species. Developing methods using this approach can be complex, subjective and costly for acquisition of the multi-spectral imagery and lidar. In contrast, deep learning models use pre-labelled images to learn the hard-to-quantify features and appearance that make objects visually distinctive to humans. With enough low-cost imagery, the models can achieve state-of-the-art classification accuracies.
For their pōhutukawa identification experiment, Grant was supplied with a huge amount of data collected by AsureQuality for MPI when they manually searched Tauranga and surrounds for pōhutukawa in 2017 and 2019. In 2017 there was little to no evidence of flowering in the imagery, but in 2019, the red blossoms covered the canopies. This provided Grant and his team with the ideal data to train and test their models on.
Using the 2019 imagery, the deep learning model could easily identify pōhutukawa based on the characteristics of their distinctive red flowers. With the imagery from 2017 (no flowers present), the model began to discern common traits of pōhutukawa that are present all year round, including their multi-leader crown shape, buds or seed capsules, and the texture and blueish hue created by the large waxy and elliptical leaves.
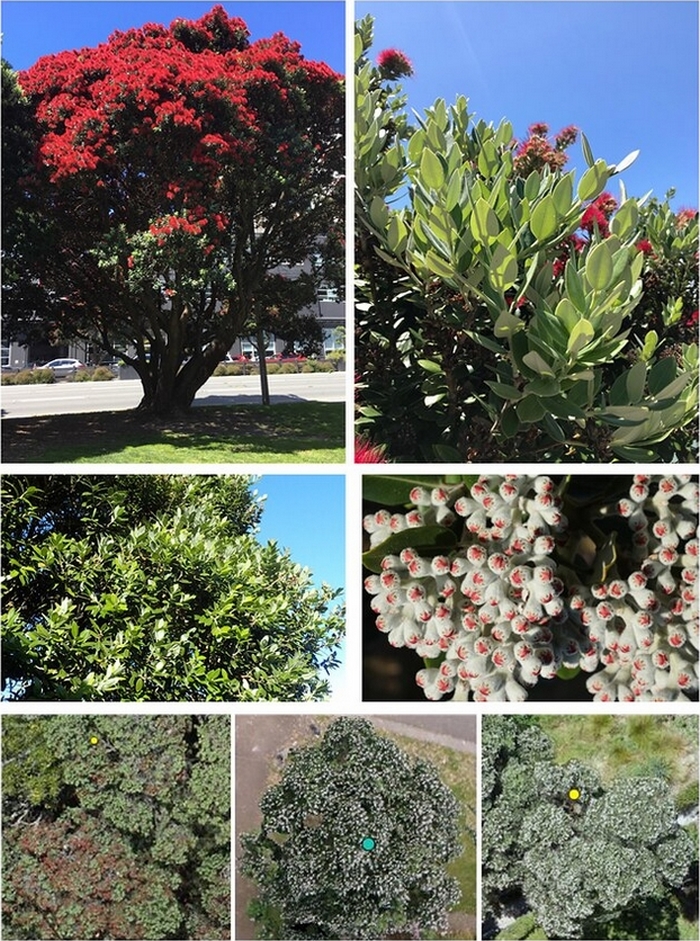
Results
When the pōhutukawa were in bloom, the deep learning model produced a near-perfect classification of 99.7 per cent accuracy (only 2 mistakes out of 569 samples), despite the level of flowering varying substantially. Without the distinctive flowering, the deep learning model still achieved 95.3 per cent accuracy despite significant differences in the appearance of the pōhutukawa.
Grant says, “Generalisability is the goal of any species classification model. We want to know if the model might be useful elsewhere in New Zealand. This is especially important for biosecurity applications where we need accurate, fast detection to manage incursions.”
“To test this, we combined datasets from 2017 and 2019 to assess the technique in real-world conditions, where flowering and imagery sources will differ. The combined model’s results were high at 98.1 per cent accuracy, suggesting there’s huge potential to generalise to real-world, large-scale biosecurity mapping applications.”
As a comparison, Grant, Alan and the team also used more traditional spectral-based remote sensing techniques to perform the same tasks. The spectral-based models did not perform as well, only detecting 88.1 per cent (with flowering), 80.0 per cent (without flowering) and 84.9 per cent (combined).
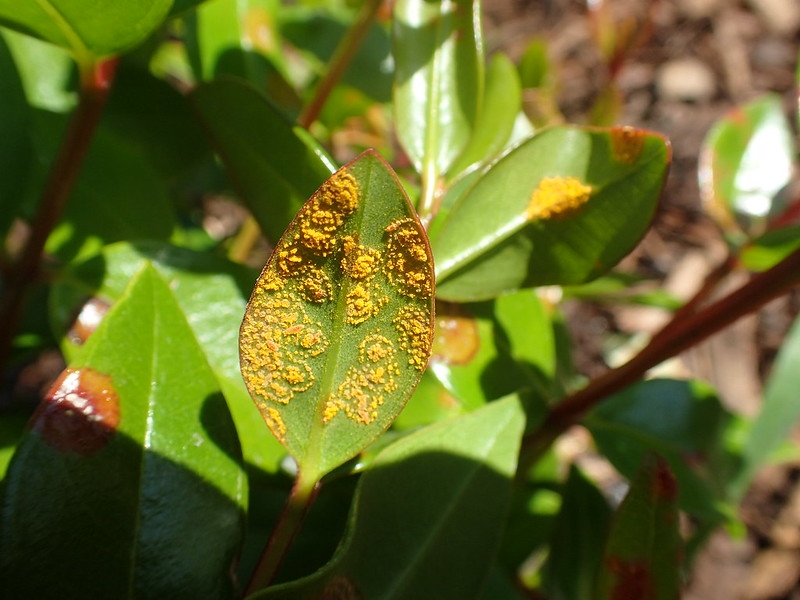
Limitations
The accuracies observed in this study were only possible with high-resolution imagery (10 cm = 1 pixel). A brief test conducted by reducing the resolution of some of the imagery showed that the accuracy of the model declined rapidly as the distinctive features of trees were lost, with 15 cm simulated imagery showing only 70 per cent accuracy.
These models also need large amounts of training data. In this case, the number of samples (1139) was very large compared to other tree identification studies, but quite low by the standards of deep learning model development. A key finding was that key plant traits, such as flowering, can be used to help identify enough trees to train a large deep learning model. Adding data from more images of the same scenes at different times, we can train a more generalisable model.
Potential for use in biosecurity responses
Grant’s experiment has proved that applying deep learning methods to identify potential pathogen hosts is possible using readily available, low-cost imagery and that it could be used to support future biosecurity efforts. The simple RGB aerial imagery required is already routinely captured over large areas of New Zealand.
With more training data and higher-resolution imagery, the deep learning approach could be expanded to a broader range of species across New Zealand. How well it performs will depend on how visually distinctive the target species are and at what resolution these features are distinctive.
The future, Grant says, is in predicting what will arrive in New Zealand next, and preparing by mapping host species before the pest or pathogen makes landfall.