New approach to predict growth of forestry tree species
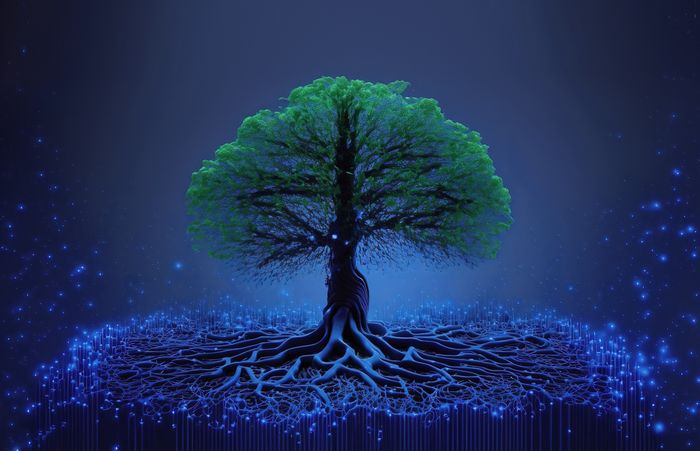
In a paper recently published in the scientific journal Forestry, the methodology was applied in a way that can help predict the carbon sequestration potential of tree species for which we currently have limited growth data.
More accurate carbon sequestration in lesser-known species can help foresters plan future plantings, more accurately predict their carbon sequestration potential and help with international climate change reporting.
The modelling approach, known as limited machine learning, is highly suitable for modelling tree species where there is limited available data. It can be used to forecast more precise forest growth and carbon sequestration to help understand how different forest types and species are affected by the changing climate.
The problem and the opportunity
New Zealand is committed on a path towards achieving a low-carbon economy by 2050 and forestry is a large part of that. To accelerate the greenhouse gas reduction process, the One Billion Trees programme aims to plant one billion trees in the country by 2028. Afforestation programmes can maximise their contribution to climate change via carbon sequestration following the right tree, planted in the right place for the right purpose ethos.
It sounds easy, but when it comes to carbon sequestration potential, quantifying it for each species is a challenge, except for radiata pine. With more than a hundred years of data in New Zealand and well-studied, long term and complete databases from many parts of the country, the carbon sequestration potential of this species is well known.
There are other less commonly planted tree species that are more suitable for long-term live carbon storage due to their longevity, size and wood density. Exotic Eucalyptus and Cypress species have desirable wood properties for construction, as do totara, and species such as mānuka offer environmental benefits due to their quick establishment and tolerance of a range of conditions.
However, there is not enough information on site productivity, growth and carbon sequestration to develop robust and precise carbon sequestration tables for less commonly planted species.
New Zealand’s current Emissions Trading Scheme (ETS) aggregates exotic species into two generalised carbon look-up tables: exotic softwoods and exotic hardwoods. Native tree species from various forest types are grouped into a single forest type – indigenous forest.
That’s just the sort of challenge that excites Scion tree ecophysiologist, Dean Meason. He says these broad species carbon sequestration tables could potentially over or underestimate tree growth and forest carbon sequestration.
“This may cause challenges for regulation of the government’s ETS scheme and international reporting. Without more precise site- and species-specific information on the suitability of individual species, there is little incentive for landowners to establish less commonly planted tree species in forests for carbon sequestration.”
This species by site planting information requires data. Forest growth data are typically captured by inventorying a large network of Permanent Sample Plots (PSPs). These are small areas, typically 0.04ha where researchers intensively measure the growth of trees over time.
The first PSPs were established in the 1920s and the PSP system is one of the most extensive data resources on tree growth, internationally. Today, the system holds data from 32,000 PSPs of which 11,500 are current and 20,500 are historic records. Most of these PSPs are for radiata pine.
While the datasets for radiata pine are comprehensive, the same quantity and quality of data is rarely available for less commonly planted species. Dealing with patchy datasets is tricky using conventional statistical approaches. Although this project was able to supplement the Scion PSP database with additional data from the Ministry for Primary Industries (MPI) Farm Management Approach (FMA) and Ministry for the Environment’s (MfE) Land-Use and Carbon Accounting System (LUCAS) databases, the tree species’ datasets were still patchy. Predicting carbon sequestration for less studied species requires a new approach beyond statistics as usual.
Machine learning
Machine learning approaches can help overcome some of the challenges when predicting tree growth from limited data inputs.
Machine learning is a type of artificial intelligence that allows software applications to become more accurate at predicting outcomes without being explicitly programmed to do so. It is a method that is especially useful at interpreting incomplete or historical information to predict the future.
In this study, a machine learning algorithm was used to predict tree diameter at breast height (DBH, 1.4 m) in response to age, stocking, site and climatic factors. This was studied for planted Mexican cypress, macrocarpa, Eucalyptus nitens, coast redwood, totara and mānuka.
Data to build machine learning models were combined from national databases, and they included stand variables, information about management, sites and climate features.
The computer models were able to predict tree DBH with high precision for the five tree species and to disentangle the many complex factors that influence DBH in these species.
This is the first attempt to utilise machine learning approaches to model tree diameter of less common planted forest tree species in New Zealand. While strictly applied to DBH in this study, this approach can be used to forecast more precise forest growth and carbon sequestration. This can help researchers and foresters to develop more accurate forest growth models and to improve current carbon look-up tables for New Zealand.
This research was funded by the MPI Sustainable Land Management and Climate Change research programme.